服务器租金应该计入哪项费用?
- 行业动态
- 2024-12-07
- 2883
服务器租金计入什么费用?这是一个涉及企业会计处理和财务管理的重要问题,服务器作为现代企业运营中不可或缺的基础设施,其租金如何计入会计科目,直接关系到企业的成本核算、税务处理以及财务报表的准确性。
一、服务器租金计入的费用科目
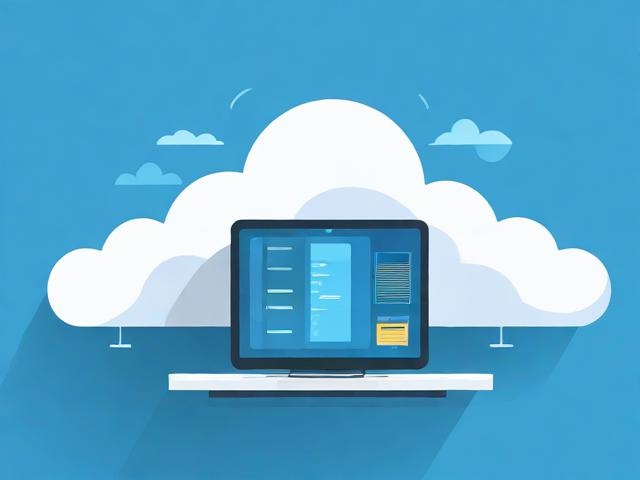
1、营业成本:如果服务器租金是直接与生产经营活动相关的费用,比如用于提供产品或服务的服务器租赁费用,那么可以计入营业成本科目。
2、办公费用:如果服务器是用于办公场所的服务器租赁费用,比如用于员工日常办公工作的服务器,那么可以计入办公费用科目。
3、折旧费用:如果企业选择购买服务器并按照一定的折旧政策进行计提,而非租赁服务器,那么服务器租金费用将计入折旧费用科目。
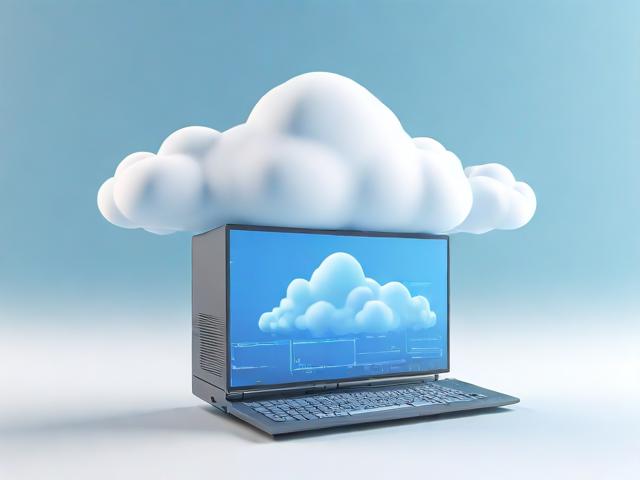
4、科研开发费用:如果服务器租金是用于科研项目或产品开发的服务器租赁费用,那么可以计入科研开发费用科目。
5、信息技术费用:服务器租金也可以计入信息技术费用科目,特别是当服务器用于支持企业的信息技术系统时。
6、租赁固定资产:如果服务器是以租赁方式获得并使用长期的,租赁费用可以记入租赁固定资产账户中的一部分,这种情况下,租赁费用会以分摊方式计入每个期间的成本。
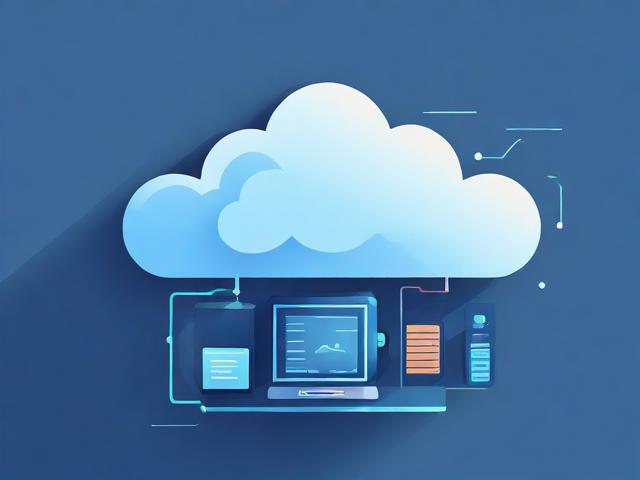
7、其他费用科目:根据公司的会计政策和实际情况,服务器租金还可能计入其他费用科目,例如与运营费用一起支付的其他费用。
8、应付账款:服务器租赁费用是公司的应付账款,因为是租用服务器的一种债务,需要在约定的时间内支付租金。
9、租赁费用:租赁费用是公司的运营费用,该费用会计入利润表中,影响公司的净利润。
二、表格展示
序号 | 费用科目名称 | 适用情况 |
1 | 营业成本 | 直接与生产经营活动相关的服务器租赁费用 |
2 | 办公费用 | 用于办公场所的服务器租赁费用 |
3 | 折旧费用 | 企业购买服务器并计提折旧的情况 |
4 | 科研开发费用 | 用于科研项目或产品开发的服务器租赁费用 |
5 | 信息技术费用 | 服务器用于支持信息技术系统的情况 |
6 | 租赁固定资产 | 长期租赁且租赁费用以分摊方式计入的情况 |
7 | 其他费用科目 | 根据公司会计政策和实际情况确定 |
8 | 应付账款 | 记录公司需要支付但尚未支付的服务器租赁费用 |
9 | 租赁费用 | 影响公司净利润的运营费用部分 |
三、相关问题解答
1. FAQs
Q: 如何确定服务器租金应计入的具体科目?
A: 应根据服务器租金的性质和用途来确定具体科目,如果服务器用于生产经营活动,则计入营业成本;如果用于办公场所,则计入办公费用等。
Q: 服务器租金是否可以税前扣除?
A: 一般情况下,服务器租金作为企业的一项正常运营成本,可以在计算企业所得税时税前扣除,但具体扣除规则需依据当地税法规定。
Q: 如果服务器租金包含维护费用,应该如何处理?
A: 如果服务器租金中包含了维护费用,并且这些维护费用能够单独区分出来,则可以将其计入相应的维护费用科目;如果无法区分,则通常一并计入服务器租金所在的科目。
四、小编有话说
服务器租金的会计处理并非一成不变,它需要根据企业的实际情况、会计准则以及税法规定进行灵活调整,在实际操作中,企业应建立健全的会计核算制度,明确各项费用的归集和分配原则和方法,以确保会计记录的准确性和合规性,企业还应加强内部控制和管理,确保服务器租金等费用支出的合理性和有效性,建议企业在处理此类问题时咨询专业的会计师或税务顾问,以获取更加准确和专业的指导。